by: Richard Lee
Summary
Learning curves is a tool to estimate recurring costs in an assembly or production process. The learning curve theory is grounded in the idea that the time required to perform a repeatable task decreases as a worker gains experience. This paper discusses two considerations of the learning curve application when developing cost estimates; (1) varying data sets, and (2) varying ranges by unit. An example data set is used in this paper to reinforce the concepts.
Introduction
Learning curve analysis is developed as a tool to estimate the recurring costs in an assembly or production process. People such as T.P. Wright (i.e., Cumulative average learning curve theory) and J.R. Crawford (i.e., Unit learning curve theory) introduced the learning curve concept. Learning curve analyses have been extensively researched and applied in numerous settings, especially within defense products.
The concept is that the time to produce a unit of output decreases at a constant rate as the cumulative output doubles. There may be several factors that contribute to the decrease of time, some of which includes:
Changes in the worker’s environment and morale;
Task simplification;
Better coordination and planning of process flows;
Design changes leading to improved producibility;
Improved communication of required information; and
Supply chain management to ensure timely availability of necessary materials.
There are two predominant theories on learning curves: Unit Theory and Cumulative Average Theory. For the purpose of this paper, the focus will be on the Unit Theory. Unit theory is defined by the following equation:
Y=A*x^b
Where
Y = the cost (man hours) of unit X to be calculated
A = the theoretical cost (man hours) of unit one (also known as T¹)
X = the unit number being estimated
b = a constant representing the slope of the learning curve (where slope = 2^b)
There are two basic principles for learning curves:
Learning takes place in a constant and predictable manner, such that when the number of units produced is doubled from n to 2n units, the number of labor hours required for the 2nth unit is less than the number required for the nth unit by a proportion, which is called the rate of learning. To illustrate this first principle, assume that the first unit cost 100,000 labor hours to produce. If an 80% curve was assumed, the second unit would require 80,000 labor hours, the fourth 64,000 labor hours, etc.
The production of each new design is unique, always starting at the beginning of the learning curve with unit number one. A new program’s learning curve is expected to be similar to a previous new program, not to current rates of improvement on a mature program. The first unit of a new program cannot be compared to the 30th or current unit of an existing program.
For the purpose of the paper, let’s consider a major acquisition defense program that has had multiple units delivered, and the objective is to estimate labor hours for future units by leveraging results from the historical learning curve analyses. In addition to performing the learning curve regressions, analysts should also take into consideration the following:
Varying data sets
Varying ranges by unit
Consideration 1: Varying Data Sets
Learning curve regressions could be performed on varying data sets, such as (1) total labor hours, (2) end use hours (i.e., total labor hours less service & support hours), and (3) end use hours less nonrecurring hours. When performing learning curve analysis, users need to be aware of the overarching methodology and the data set used to develop the estimate. For example, if an estimate was developed using end use hours, it would NOT be appropriate to apply a learning curve rate derived using total labor hours.
Figure 1 shows an example of learning curve regressions using two different data sets, (1) end use hours, and (2) end use hours less non-recurring. Both data sets are from the same program, but normalized differently. From Figure 1, the unit learning curve rates show different results.
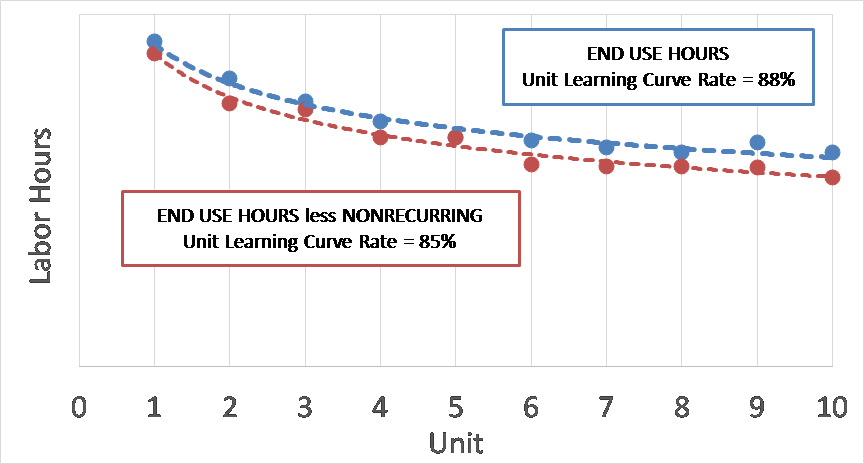
Which learning curve rate should be used to estimate units 11 through 20? As stated above, the overarching methodology will have to be considered. If the base estimate is derived using end use hours only, then the learning curve rate of 88% should be used. If the base estimate is derived using end use hours less non-recurring, then the learning curve rate of 85% should be used.
Consideration 2: Varying ranges by unit
Learning curve regressions could also be derived based on varying unit ranges. Ideally, all data would be used for the learning curve analyses, but there may be legitimate rationale to remove certain units. Using the same example above, assume units 1 through 5 reflects the first batch and units 6 through 10 reflects the second batch for the program. If there are major differences in design / processes between the two batches, there may be consideration of only using the learning curve rate derived from units 6 through 10. As the analysts, an approach could be to simply calculate all possible outcomes from the data set received to help inform decision makers all potential options.
Figures 2 through 4 show the results from performing the learning curve regressions using varying unit ranges from the end use hours as reflected in Figure 1. Figure 2 shows the learning curve rates using varying unit ranges, Figure 3 shows the R² values using varying unit ranges, and Figure 4 shows the percent delta between the actual first unit and the theoretical first unit (i.e., T1) using varying unit ranges. As the results show, as the initial units are removed from the regressions, the learning curve rate increases (i.e., learning curve becomes ‘flatter’), the R² decreases and % delta from T1 increases (i.e., both signs showing that the learning curve fit is becoming ‘worse’).

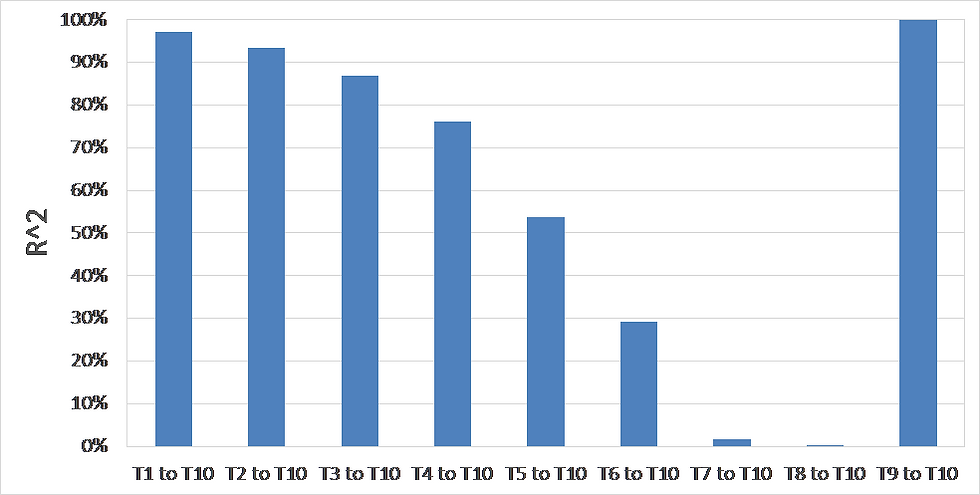
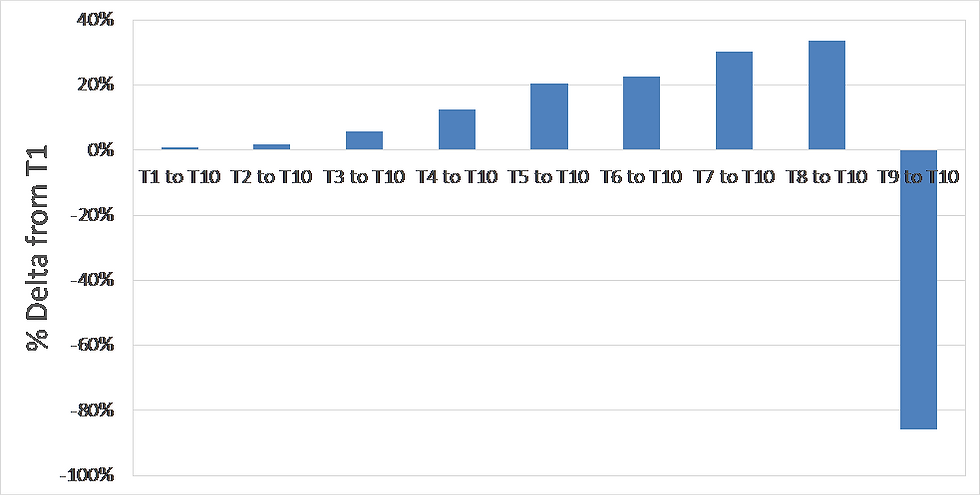
Conclusions
The learning curve theory is founded on the concept that the if a task is performed repeatedly, then less time will be required for subsequent units as a worker gains experience and improves efficiency. This paper discusses two considerations of the learning curve application when developing cost estimates; (1) varying data sets, and (2) varying ranges by unit. When considering varying data sets, be sure to understand the overarching methodology and the raw data set used for the base estimate. Analysts should be consistent between the development of the cost estimate and the application of the learning curve rate. As for the varying ranges by unit, statistics show that performing regressions using the entire data set is better than removing initial units. However, there may be potential arguments to remove earlier units.
Pantheon Integrated Solutions, Inc. is experienced in learning curve analyses for cost estimates, contract negotiations, and other applications. Organizations that seek to estimate future capabilities or to negotiate contract costs for acquisition programs should contact Pantheon Integrated Solutions, Inc. to discuss the appropriate learning curve methodology.
Comments